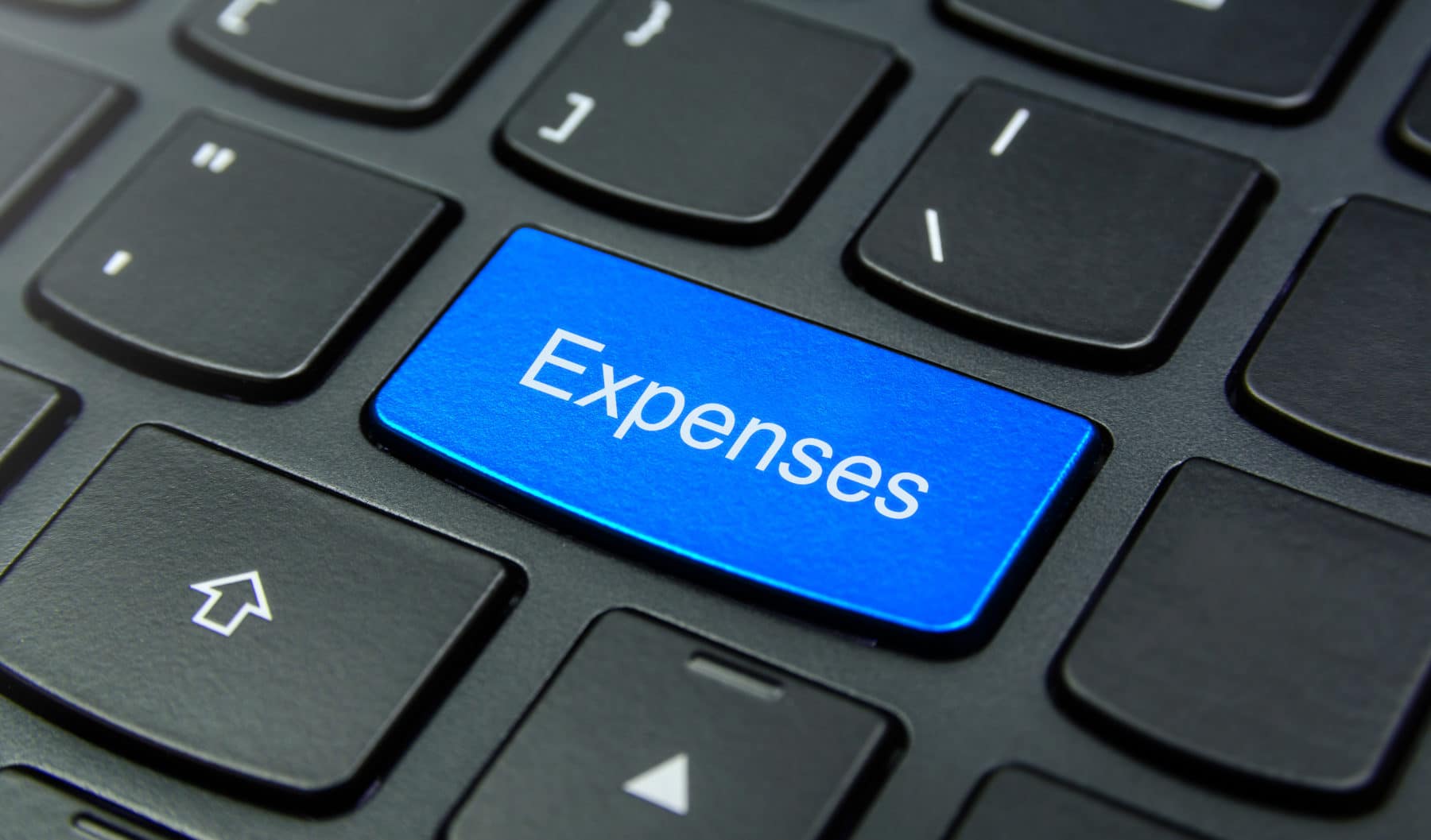
Expenses – The Wise and the Mighty
July 31, 2019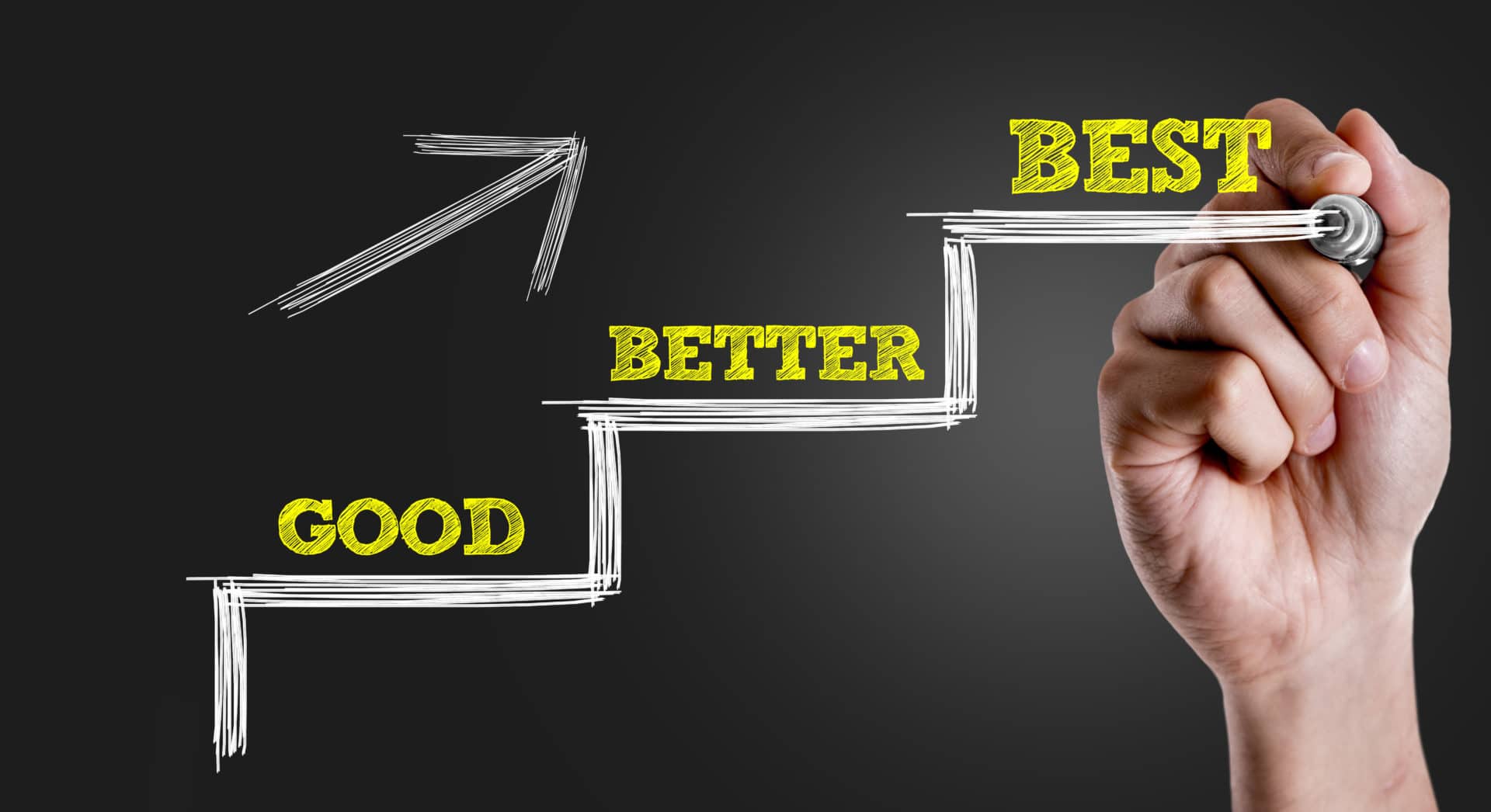
Tips for Qualifying a Speech to Text Transcription Service
August 22, 2019The Data Cube Conundrum in Academic Research: If the data is wrong, can the model ever be right? Consider methodology and an academic transcription service.
Academic research is a challenging, but necessary, undertaking by any professional academic or researcher. The primary goal is to contribute to one’s respective field by answering important questions and gaining the knowledge to answer them through experimentation, intensive study, and methodological rigor. Quantitative and qualitative data plays a critical role in research—done properly, any data used should be evaluated for its quality at every stage—from initiation, analysis, to conclusion. The findings must be able to present information that is credible, defendable, and tractable. With such a complex and sometimes time-lengthy process in research, data is like a multi-sided cube with each dimension constituting the study and analyses.
What we can’t ignore? Methodology and study design. What helps the quality of the data? An academic transcription service.
In research, data should satisfy the requirements of the established study design and a checklist for ensuring it is properly collected and processed. So, let’s consider what data should meet:
1. Data Collection: How data is collected is critical to the study design. Specifically, is it adequate (enough to continue with the study and analyze), duplicative, or erroneous resulting in affected reliability, integrity, and validity? How you are capturing or recording data is critical.
2. Data Storage: As data is collected, how is the data stored? Is it secure? To what type of encryption security is the data subjected? This is critical to ensuring its integrity and validity. In some fields, such as Biology crossed with the Healthcare industry, data must meet HIPAA compliance. This means appropriate electronic storage of medical transcripts, voice dictations, video, and similar data; specifically, Protected Health Information (PHI).
3. Data Quality: Data should be reviewed for noise, as in data that is not relevant to the data collected towards the study objective. Furthermore, errors generated from incompleteness, unnecessary duplications, incorrectly processed calculations and data transformation, and more should be identified. If you think you will not have these kinds of errors in your data set(s), that is an erroneous assumption.
At Athreon, accuracy is upheld by incorporating rubrics, automation, human intervention in quality assurance, and a reinforcing feedback system. Audio data, for example, rarely just captures the spoken word without ambiguity. Athreon’s objective (met time-and-time again) is to ensure that data is more accurate than any speech-recognition mobile app or software available. Furthermore, we ensure data handling is based on HIPAA and the FBI’s Criminal Justice Information Services (CJIS) requirements. This shields researchers from high-technology software and hardware costs.
Following data collection, analyzing data and modeling takes on other dimensions of complexity. At every stage, assumptions should be further examined or properly defended with sound reasoning. Other dimensions of modeling and analysis consider:
4. Model Representation: As said by Massachusetts’s Institute of Technology’s System Dynamicists, every model is wrong. Why? Models are constructed from our mental databases and models based on perception, beliefs, and continuous calibration with human logic and reasoning can be flawed. That said, we should rigorously and principally seek to solve the problem or question the model and study was designed to answer. The model should strive to accurately describe the behavior(s) from the data collected. If the data is not reliable, then the model is impacted. With unreliable data, your better understanding of the problem or question will be unclear and forecasting uncertain.
5. Data Noise: There will never be a model and its data without the presence of noise. The reason being is that the real world consists of a variety of data existing and generating in a continuum. There are no ends or silos when understanding the data lifecycle. Statistically, noise may be thought of as the error variable. In other words, this is data that is not relevant to the study or model or the measurable gap to legitimate errors.
6. Forecasting and Predictive Capabilities: Forecast models are based on historical and sometimes probabilistic-type data. Unfortunately, such models could have a degree of error from the onset, because it is not dynamic or in real time. Similarly, predictive type of modeling is based on historical data, mathematical, and computational modeling. At the core, if the data is not sufficient for training or testing, then reliability becomes a concern.
7. Analytical Methods: Depending on the toolset of choice—such as a programming language, be it Julia or Python, Mathematics, System Dynamics, Deep Learning, Machine Learning, and more—analysis and computation will transform data into new data. Ongoing quality checks are necessary to ensure that data integrity is intact, which also influences model integrity and its results.
8. Model Validity: Ensure that your model(s) can be reproduced and supported by succinct documentation.
9. Criticism: When possible, share your models and data with your peers and the world. Invite questions because challenges only contribute to your learning rate and experience.
And when it comes to publishing your work or getting the attention of your most respected reviewers, laying out a path on how to collect and manage your data for even better analyses and modeling can make all the difference.
Most of all, have courage and resilience in your work and presentation. Exemplified by the greatest, be it field founders and Nobel laureates alike, you could be the only one in the room defending or upholding your model(s) and findings. And that’s a start to making great contributions ahead. There is always an opportunity to learn!
If you would like to know how Athreon can help you with your academic research efforts or projects, contact us today for a consultation and demonstration of our academic transcription service. We will help you succeed!